Description
- Review and discuss type I and Type II errors associated with hypothesis testing.
- Review and discuss the difference between statistical significance and practical significance..
- Describe the common elements present in all hypothesis tests.
POSTS REPLIES
1)1. Hypothesis testing is used to validate hypothesis statements in a research project. Hypothesis testing is often used for hypothesis discovery because it helps the researcher test hypotheses that may not be considered plausible. Hypothesis testing is also helpful for testing hypotheses that appear to require substantial evidence. Type I errors involve incomplete data or assumptions. The purpose of this type of error is to test ideas that contradict a hypothesis. In addition to hypothesis testing, people may also test for Type II errors when data does not provide enough information to be made reliable based on an observed database (Chris, 2019). If people do not have enough evidence to build a solid hypothesis, one must take additional measures to explain the data. Examples of a Type I Error Type I errors arise from failing to identify multiple potential sources of error. The purpose of a type I error is to make sure an estimated error is a reasonable estimate. For example, it is more accurate to say they would have overestimated the time taken to do an experimental task. There is no guarantee that all possible factors would have contributed to a less than an optimal assessment of statistical significance. People may also find that they have made an erroneous estimate of the likelihood of an alternative explanation when they have data of interest but no other known source of uncertainty. Types I errors are not error-prone or challenging to diagnose. One can use these types of errors to help determine whether a finding in a scientific study has enough evidence to warrant further analysis. Type I errors can be helpful to help clarify their conclusions in future studies and to prevent confusion (Chris, 2019).
Examples of Type II Errors: Type II errors are essential for researchers working on complex statistical models. To deal with types of errors, people must develop a strategy to evaluate and decide what is most important. For example, a study of sexual behavior may be to develop a hypothesis that would better explain the observed behavior. It may also be that people are trying to determine whether a behavior is genuinely stable over time, which may mean they need to use more or less information than they initially reported (Chris, 2019).
The following examples describe types of Type II errors: These are errors that have been encountered when testing a hypothesis. They can cause people to fail to confirm or reject a hypothesis. People often see these types of errors as the results of an incorrect assumption. When people know what to do with a Type I Error, and they are confident it will not happen again, people usually ignore it. With a Type II Error, people are more likely to miss the significance of a single data point. Type I errors are caused by an incorrect interpretation of the data. Hypothesis tests cannot be considered Type II errors because they are not intended to help them discover whether the hypothesis is true or false (Chris, 2019).
2. Significance (S) indicates the strength of the relation between two independent variables and denotes either the degree of independence of the relation or the number of degrees of freedom included. Practical Significance (PS) is the number of degrees of freedom that are included. In other words, the more the variables are included, the better the relationship. Statistical significance describes the importance of a result based on sample size and population of interest. Statistical significance is a measure of whether or not the data and analysis findings provide a significant difference. In other words, whether the results meet a threshold level of statistical significance. The more meaningful the results, the greater the statistical significance. A more accurate definition of statistical significance is that the results should be significant if one must make assumptions about the data to interpret the results (Andrei, 2021).
An important distinction between statistical significance and practical significance involves the nature of the information being sought. In statistical terms, the information may be simple or complex. More often than not, the information can be used for many other applications besides statistical or practical analysis. In practice, many times, those applications may be more specific. It makes it all the more important for researchers to recognize the term statistical significance when they use that term. In addition, people seeking, understanding, or studying this kind of information often prefer to use the phrase very little evidence when describing the significance of their data and analysis. The idea that statistical significance means rejecting a hypothesis or not found is not precisely pragmatic. It has often been used in science writing and many discussions around this issue. A statistical significance is a quality of evidence that indicates the presence of a new finding. It will be the amount of data that is needed to prove the significance. Therefore, the amount of data needed to prove the significance of a finding will be more significant with statistical significance than practical significance (Andrei, 2021).
3. To summarize, this section reviews common elements that appear in the various Hypothesis Tests. Each of these common elements has a short explanation for this term, along with a link to a video discussion on those elements. Characteristics of an Outcome To summarize, a Hypothesis Test contains an example of a behavior, which could be one way to describe the outcome that it tests. All Hypothesis Tests perform similar checks to compare a hypothesis against known reality (Chris, 2019). These checks include examining the validity of a subject’s predictions using an empirical method and testing whether each Hypothesis Test has evidence against its subject. In addition, a Hypothesis Test may analyze information from one hypothesis to determine if the same underlying hypothesis can be tested with a different method. For example, the authors found that Hypothesis tests typically perform less with the use of statistical analysis when used with the use of a larger sample. More research is needed to determine if using the statistical method is a factor in decreasing testing effectiveness for Hypothesis Tests (Chris, 2019).
Reference
Andrei, A. C. (2021). Statistical significance: Is there a way out of it?. The Journal of Thoracic and Cardiovascular Surgery, 161(4), 1377-1378.
Chris.s, (2019).Basic statistics _ tales of distributions.
2) Key terms and concepts in Hypothesis Testing
:
- Null hypothesis: Null hypothesis is a statistical hypothesis that assumes that the observation is due to a chance factor. Null hypothesis is denoted by; H0: ?1 = ?2, which shows that there is no difference between the two population means.
- Alternative hypothesis: Contrary to the null hypothesis, the alternative hypothesis shows that observations are the result of a real effect.
- Level of significance: Refers to the degree of significance in which we accept or reject the null-hypothesis. 100% accuracy is not possible for accepting or rejecting a hypothesis, so we therefore select a level of significance that is usually 5%.
- Type I error: When we reject the null hypothesis, although that hypothesis was true. Type I error is denoted by alpha. In hypothesis testing, the normal curve that shows the critical region is called the alpha region.
- Type II errors: When we accept the null hypothesis but it is false. Type II errors are denoted by beta. In Hypothesis testing, the normal curve that shows the acceptance region is called the beta region.
- Power: Usually known as the probability of correctly accepting the null hypothesis. 1-beta is called power of the analysis.
- One-tailed test: When the given statistical hypothesis is one value like H0: ?1 = ?2, it is called the one-tailed test.
- Two-tailed test: When the given statistics hypothesis assumes a less than or greater than value, it is called the two-tailed test.
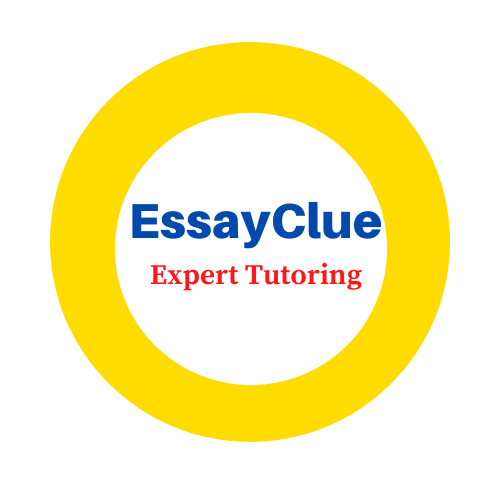
Get FAST homework help. Place a similar order and get a 15% Discount for your first three orders. We have a team of professional tutors to help you with any assignment regardless of the deadline. Contact us for immediate Homework Help.